by Yun-Ning Hung
Motivation
Music source separation performance has been improved dramatically in recent years due to the rise of deep learning, especially in the fully-supervised learning setting. However, one of the main problems of the current music source separation systems is the lack of large-scale datasets for training. Most of the open-source datasets either have limited size, or the number of included instruments and music genres is limited.
Moreover, many existing systems focus exclusively on the problem of source separation itself and ignore the utilization of other possibly related MIR tasks which could lead to additional quality gain.
In this research work, we leverage two open-source large-scale multi-track datasets, MedleyDB and Mixing Secrets, in addition to the standard MUSDB to evaluate on a large variety of separable instruments. We also propose a multitask learning structure to explore the combination of instrument activity detection and music source separation. The goal is that by training these two tasks in an end-to-end manner, the estimated instrument labels can be used during inference as a weight for each time frame. We refer to our method as instrument aware source separation (IASS).
Model structure

Our proposed model is a U-net based structure with residual blocks instead of CNNs at each layer. The reason for choosing the U-net structure is that it has been found useful in image decomposition, a task with general similarities to source separation. The residual block allows the information from the current layer to be fed into a layer 2 hops away and deepens the structure. A classifier is attached to the latent vector to predict the instrument activity. Mean Square Error (MSE) loss is used for source separation while Binary Cross-Entropy (BCE) loss is used for instrument activity prediction.
Instrument weight
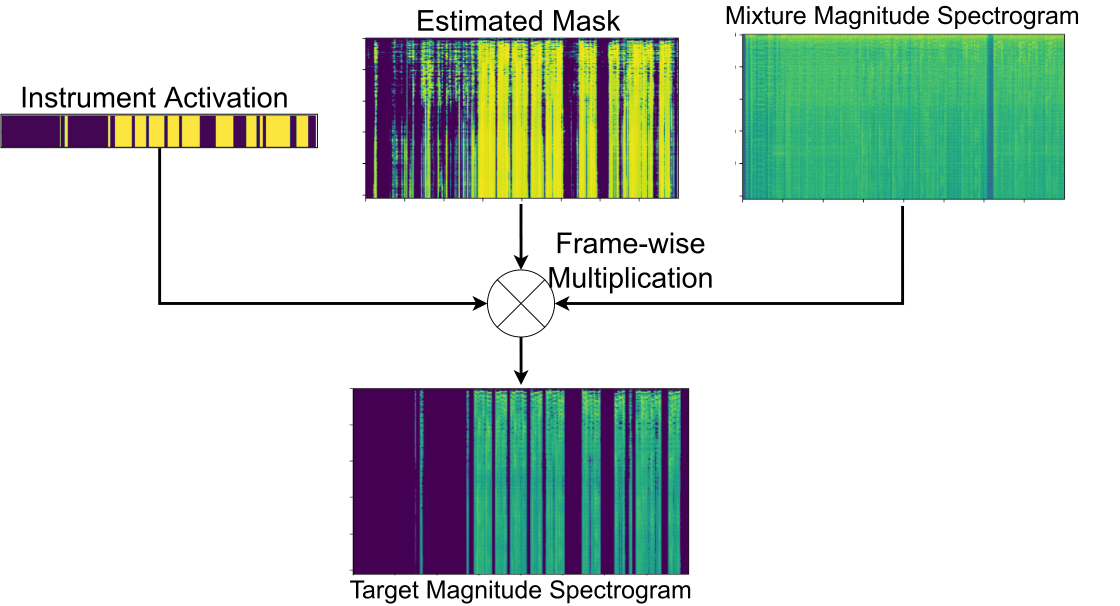
We use instrument activation as a weight to multiply with the estimated mask along the time dimension. By doing so, the instrument labels are able to suppress the frames not containing any target instrument. The instrument activation is first binarized by using a threshold. A median filter is then applied to smooth the estimated activation.
Experiments & Results
We compare our model with baseline Open-Unmix model. Both models use mixture phase without any post-processing. We train and evaluate both models on two datasets, MUSDB-HQ dataset and the combination of Mixing Secrets and MedleyDB.
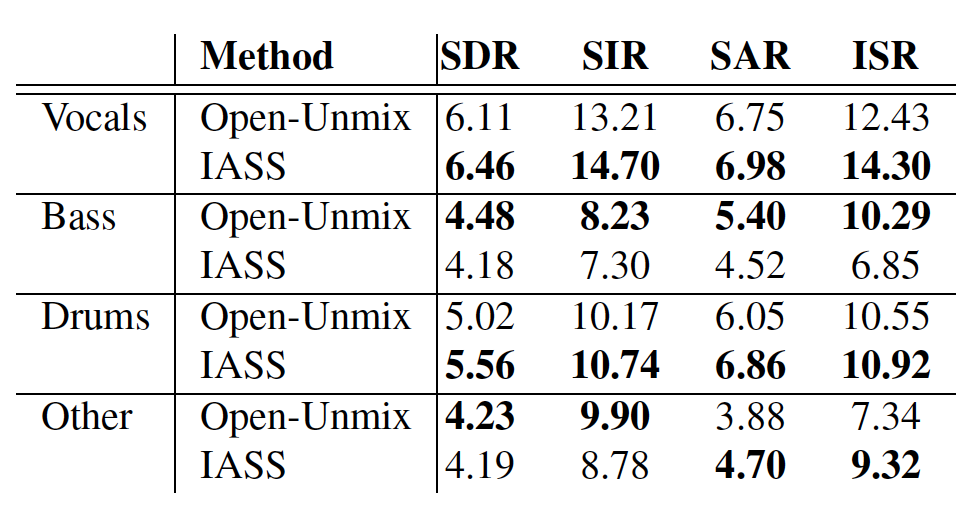
The result shows that when training and evaluating on the MUSDB-HQ dataset, our model outperforms the Open-Unmix model on ‘Vocals’ and ‘Drums’, performs equally on ‘Other’, and slightly worse on ‘Bass’. This might be because ‘Bass’ is likely to appear throughout the songs; as a result, the improvement of using the instrument activation weight is limited.
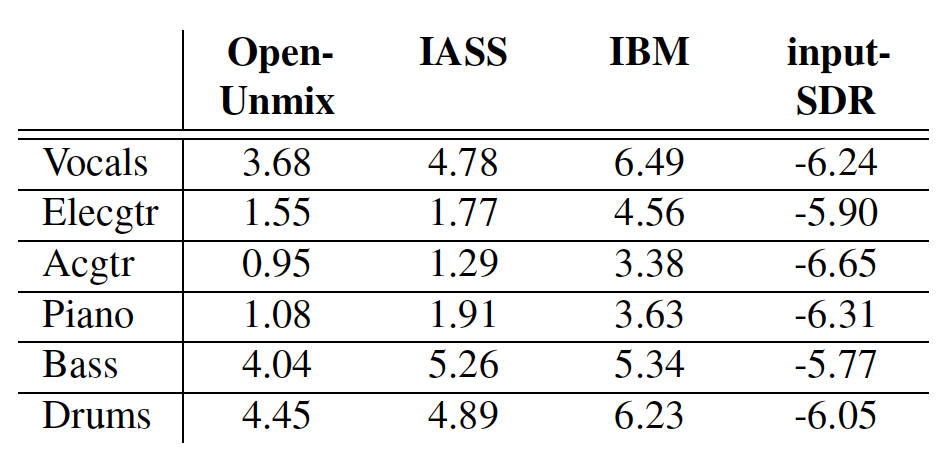
Figure 4 summarizes the results for the combination of the MedleyDB and Mixing Secrets datasets. The Ideal Binary Mask (IBM) represents the best-case scenario. The worst-case scenario is represented by the results for input-SDR, which is the SDR score when using the unprocessed mixture as the input. We can observe that our model also outperforms Open-Unmix on all the instruments. Moreover, both models have higher scores on ‘Drums,’ ‘Bass,’ and ‘Vocals’ than on ‘Electrical Guitar’, ‘Piano’, and ‘Acoustic Guitar’. This might be attributed to the fact that ‘Guitar’, ‘Piano’, and ‘Acoustic Guitar’ have fewer training samples. Another possible reason is that the more complicated spectral structure of polyphonic instruments such as ‘Guitar’ and ‘Piano’ make the separation task more challenging.
Conclusion
This work presents a novel U-net based model that incorporates instrument activity detection with source separation. We also utilize a larger dataset to evaluate various instruments. The result shows our model achieves equal or better separation quality than the baseline model. Future extension of this work includes:
- Increasing the amount of data by using a synthesized dataset,
- Incorporating other tasks, such as multi-pitch estimation, into our current model, and
- Exploring post-processing methods such as Wiener filter, to improve our system’s quality.
Resources
- The code for reproducing experiments is available on our Github repository.
- Please see the full paper (to appear in ISMIR ’20) for more details on the dataset and our experiment.